The B2C Premium
Within my REIT coverage, I’ve often found it useful to separate out the consumer-facing property types like residential and self-storage, and think about why they’ve been such consistent long-term outperformers relative to “B2B” sectors like office.
If we set aside the extreme trends of the past few years, and just look at their pre-Covid outperformance — should we expect similar outperformance in the future? Or were there B2C advantages in the past that that may now be used up?
The one I’ll start with today is revenue management. And along the way, I’ll try to engage with the recent ProPublica investigation of YieldStar. I’m not convinced by their case for “algorithmic collusion,” and in a sense, it’s the opposite of the diminishing-returns story that I’m about to tell. But I think they’re picking up on some real issues that investors should be thinking about too.
Phase One
In 2019 I was writing about hotel loyalty points, and reading some old econ papers about their predecessor programs in the airline industry. One of them included a passing remark about housing that has stuck in my head:
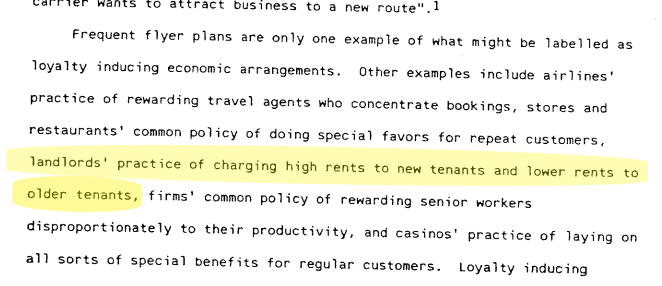
Was it really still true in 1987 that renewing tenants were all getting below-market offers? I don’t know, but it certainly wasn’t true by 2019 — at least, not for large and sophisticated landlords like the apartment REITs. In fact, I can think of only one REIT with a stated policy of capping renewal offers at market, rather than trying to squeeze out a little more.
So how did the industry reach this point of competing to punish customers for loyalty? And why haven’t we seen the same effect in B2B property types?
We could talk about capex and other variables here, but I think the most obvious differences are in the game theory of the rent negotiation itself. Compared to a commercial tenant with a good broker, the average individual is simply in a worse negotiating position relative to their landlord.
And it’s easy to see how some of the relevant frictions could apply more to a renewing tenant than a new one. For example, a resident faces higher costs of moving than the landlord would incur to turn the unit and replace them — along with higher uncertainty around those costs, and often less capacity to finance them. But for a new renter, much of this is either a sunk cost if they’re already committed to moving, or an option if they’re not. So one benefit of automated revenue management is that it helps the landlord to fully exploit these advantages over existing tenants at renewal.
Of course this isn’t the only thing the software does, or even the first. In fact, I think the early years of implementation were more focused on pricing vacancy, which is a more direct analog to the travel sectors where this software was first developed. So that may have been more about maximizing the landlord’s informational edge around real-time demand indicators like leasing traffic — and some of those gains may have come more from competing landlords than from tenants.
But all these frictions are finite. And when you get to the point where you’re consistently achieving mid-single digit renewal spreads, even as market rents flatten out — which is where some apartment REITs were in the late-cycle pre-Covid environment — it may be a good indicator that you’ve captured all the low-hanging fruit, and you’re running out of steam.
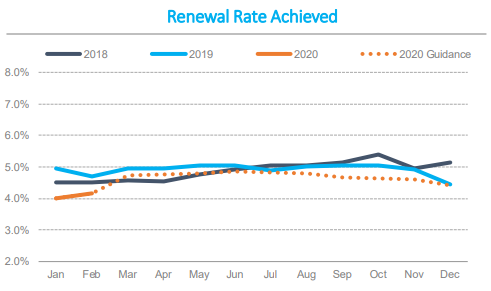
The “AI” Trap
The problem that I’m building up to is not unique to real estate. You can see a version of it in every other industry that’s adopted yield management, and it’s closely related to the flawed CAC/CLTV math that helped drive this most recent tech bubble. In its most general form, it’s been one of the most effective tricks that investors have fallen for in the past decade.
The trick is to use the rhetoric of algorithms, “AI” and “data science” to make this kind of diminishing return trend look more linear. This is increasingly how revenue “optimization” is sold to corporate management, it’s how management sells it to investors, and it’s how investors sell it to themselves.
And for what it’s worth, I don’t think real estate has fallen for it nearly as hard as some other sectors. For most of the past two decades, I think landlords implementing yield management have had a pretty good feel for what it’s doing. It’s only in recent years that some of the REITs have started to sound fuzzy about it, and slip into some of the “AI” rhetoric that I’ve learned to be skeptical of in tech, retail and elsewhere.
Again, the problem is not that “data science” doesn’t work. The problem is when companies lose sight of how it’s working, and start to invest more as the marginal returns tail off.
But the solution is simple. Whenever you hear “optimization,” think “diminishing returns.” And when the returns are not tailing off, make sure you understand exactly where else they’re coming from, and whether it’s worth it.
Phase Two
Where else could they be coming from? The next phase is the interdependent, market-level effects of widespread adoption — corresponding to the widely repeated view that yield management works better “when everyone else is using it.”
To be clear, “interdependent” is a long way from collusive or illegal. But this does suggest there’s a legal ceiling on how far this effect can go, and we’re dealing with another finite opportunity that may soon be exhausted.
Or has it gone too far already? That’s the charge in this ProPublica article:
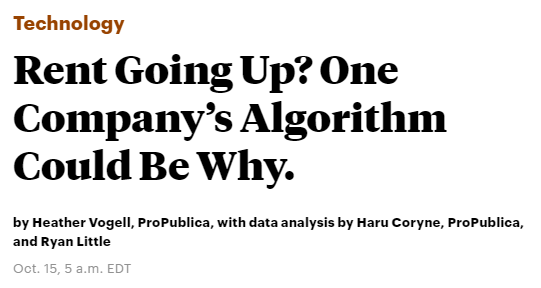
Like many an exposé, this one is dropping a legal claim into a heated policy debate, and it’s understandable that some of the initial responses are in policy terms. If your first reaction to the above headline is that most excess rent growth is driven by undersupply, most of the US rental market is too competitive for any kind of price-fixing, and our best tool for housing affordability is more new housing — I agree with all of that.
But it’s not really engaging with the specific allegations in the article and ensuing class-action suit, or the legal standard they’re appealing to:
Imagine a group of competitors sub-contracting their pricing decisions to a common, outside agent that provides algorithmic pricing services. Each firm communicates its pricing strategy to the vendor, and the vendor then programs its algorithm to reflect the firm’s pricing strategy. But because the same outside vendor now has confidential price strategy information from multiple competitors, it can program its algorithm to maximize industry-wide pricing…
So I’ll try to be specific. First, I think the ProPublica team may have a good point about the dynamics of the past few years. When you have an extreme demand shock that pushes vacancy down to frictional levels, it’s not implausible that widespread yield management in a given submarket could amplify — not cause, but amplify — the resulting spike in rents. This Twitter thread is one good account of how that could happen:
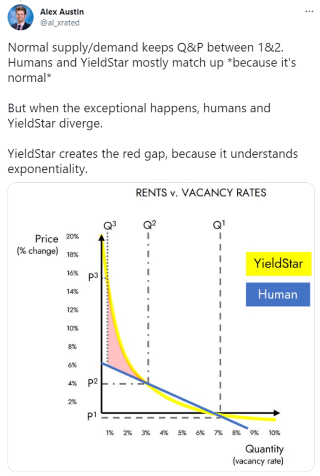
But like him, I don’t see how it requires a hub-and-spoke conspiracy. In other words, you’d get the same amplification even if you broke up YieldStar into five independent software companies.
Are these rent spikes still a legitimate cause for public anger? Absolutely. And even if there’s no antitrust remedy, other regulation may be warranted, like some of the emergency rent caps during Covid. In the long run, the most effective response would be to subsidize even more new supply, so that the next demand shock can’t have the same effect.
There are other details in this lawsuit that I won’t get into, and it’s certainly still possible that YieldStar was doing something wrong. I’m not defending them, and I’m not defending anyone else’s system either. But for our purposes here, let’s give them all the benefit of the doubt, and assume there was nothing nefarious going on in the pre-Covid period we’re focused on — and that even the non-nefarious forms of “interdependence” contributed only a small amount of market rent growth.
Even so, small amounts are what we’re talking about, right?
So let’s say that revenue management contributed 50bps annually to apartment REIT rent growth over that pre-Covid decade. And let’s say it was mostly driven by the dynamics in phase one, and as they ran out, they were supplanted by this boost from more widespread adoption.
Now that the juice is mostly squeezed from both — even without a regulatory response that claws some of it back, this is 50bps of incremental B2C-over-B2B rent growth that we shouldn’t expect to keep getting.
Unless there’s a third trick?
Phase Three
Again, we don’t have to guess, because we’ve already seen it in other sectors, and we’re starting to hear it from the REITs. Phase three of the “optimization” narrative is personalization, and increasingly individualized targeting — not just in terms of rental rates, but cross-selling other services and amenities.
But from what I can tell, most of the results in these other sectors have not been impressive. And this makes sense, because it’s really a retread of phase one, right? When you strip out all the marketing jargon, it’s just going back to the same dry well and digging even deeper. The low-hanging fruit has already been picked.
And remember that collusion is not the only way that algorithmic pricing has gotten non-real estate companies into legal or reputational trouble. Another minefield is inadvertent bias and discrimination, and it’s one that we have to be especially sensitive to in housing.
So when I hear the REIT “data science” routine starting to include more individual profiling of residents, I just have to wonder how the risk/reward could possibly make sense. I mean, do you really want to wind up sending different renewal offers to two otherwise comparable units in the same building, based on scoring the residents with a black box “AI” that you can’t fully audit? For another 20bps of revenue growth? As a public company? Really?
In part two, I’ll look at self-storage and hotels on this same framework, and contrasting them with apartments will lead us to some larger B2C drivers. In the meantime, hopefully it’s clear what I’ve been trying to do here. I’m not using these systems at the property level, and I’m not aiming for a comprehensive account of everything they do. I’ve also broken down market power in an informal way that would probably not satisfy an economist or an antitrust lawyer, and these “phases” are more overlapping and intertwined than I’ve made them sound.
But from an investor perspective, this is the level of explanation that we should be looking for. And again, this is not just a point about real estate. We should never just accept that any company’s incremental investments in data, analytics and “optimization” will keep paying off. We need an intuitive understanding of what they’re doing, and how long it will last.